Machine learning, a subset of artificial intelligence, has emerged as a transformative force in the healthcare industry. By using algorithms to identify patterns and make predictions based on large datasets, ML enables healthcare professionals to make data-driven decisions and unlock new possibilities in patient care.
From diagnosis and treatment planning to drug discovery and telemedicine, machine learning applications are rapidly changing the way healthcare providers approach patient care, making it more accurate, personalized, and efficient.
Importance of ML in Modern Healthcare Systems
The incorporation of machine learning into modern healthcare systems is essential for addressing the increasing complexity of medical conditions and the growing demand for efficient care. By automating routine tasks, ML can reduce the workload on healthcare professionals, allowing them to focus on more critical aspects of patient care.
Furthermore, ML enables the integration of vast amounts of data, including electronic health records, genomics, and medical imaging, to provide insights that were previously unattainable. As a result, ML-driven healthcare systems are better equipped to detect diseases early, develop targeted treatment plans, and monitor patient progress, ultimately leading to improved patient outcomes and reduced healthcare costs.
Scope of the Article
This article aims to provide an overview of the benefits and use cases of machine learning in healthcare. We will explore how ML is revolutionizing various aspects of healthcare, such as diagnostics, personalized medicine, cost reduction, drug discovery, and telemedicine.
Additionally, we will discuss specific use cases in areas like oncology, cardiology, neurology, mental health, and infectious diseases. Finally, we will touch upon the challenges and limitations that must be addressed for the successful integration of machine learning into healthcare systems.
Benefits of Machine Learning in Healthcare
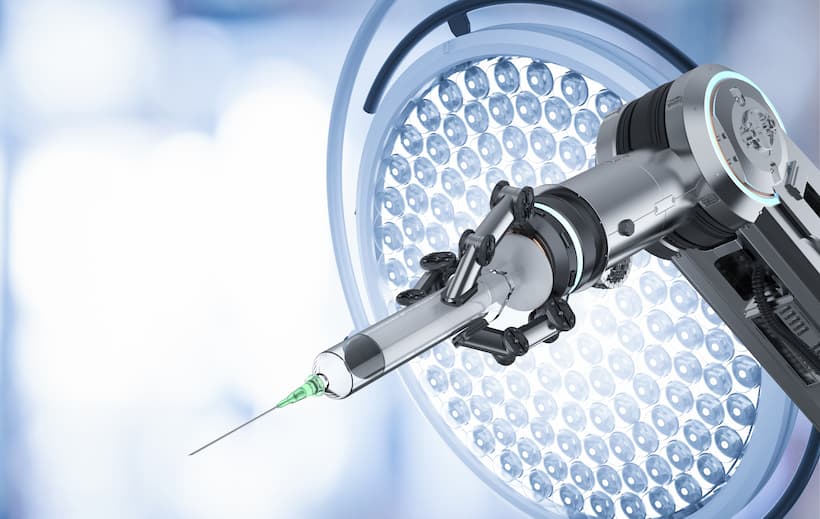
Machine learning has rapidly become a key driver of innovation in healthcare, offering a myriad of benefits that enhance patient care and streamline medical processes. By harnessing the power of ML algorithms, healthcare providers can achieve improved diagnostic accuracy, develop personalized treatment plans, reduce overall costs, accelerate drug discovery, and advance telemedicine capabilities. These transformative applications promise to revolutionize the healthcare industry and improve patient outcomes on a global scale.
Improved Accuracy in Diagnostics
Machine learning enhances diagnostic accuracy by facilitating advanced image analysis, early disease detection, and data-driven decision-making, ultimately leading to better-informed treatment decisions and improved patient outcomes.
Enhanced Image Analysis
Machine learning algorithms are capable of analyzing medical images with greater precision and speed compared to traditional methods. By leveraging deep learning techniques, ML can identify subtle patterns and features in imaging data, such as X-rays, MRIs, and CT scans, which may be missed by human experts. This enhanced image analysis leads to more accurate diagnoses and better-informed treatment decisions.
Early Detection of Diseases
Machine learning can process and analyze vast amounts of data from various sources, including electronic health records, genetic information, and wearables, to detect early warning signs of diseases. By identifying patterns and trends indicative of health issues, ML enables healthcare professionals to intervene earlier, improving patient outcomes and preventing the progression of potentially life-threatening conditions.
Personalized Treatment Plans
Machine learning enables the development of personalized treatment plans by analyzing complex patient data, tailoring treatments to individual needs, and improving the effectiveness and safety of medical interventions.
Analyzing Patient Data
Machine learning can analyze complex and diverse patient data to provide personalized treatment recommendations. By processing information such as medical history, genetic makeup, and lifestyle factors, ML algorithms can identify the most effective treatment options for individual patients, taking into account their unique circumstances and needs.
Tailoring Treatments to Individual Needs
By leveraging the insights gained from patient data analysis, healthcare providers can design treatment plans specifically tailored to each patient’s unique needs. This personalization not only increases the effectiveness of treatments but also minimizes the risk of side effects and complications, ultimately leading to improved patient satisfaction and outcomes.
Reducing Healthcare Costs
Machine learning contributes to reducing healthcare costs by streamlining administrative tasks, optimizing resource and staff allocation, and improving hospital operations and overall efficiency.
Streamlining Administrative Tasks
Machine learning can automate time-consuming and repetitive administrative tasks, such as appointment scheduling, billing, and insurance claims processing. By reducing the burden on healthcare staff, ML allows them to focus on more critical aspects of patient care, improving efficiency and reducing overall healthcare costs.
Optimizing Resources and Staff Allocation
Machine learning can analyze patient flow, equipment usage, and staff performance to optimize resource allocation and improve hospital operations. By predicting patient demand and identifying areas for improvement, ML can help healthcare facilities reduce wait times, minimize resource waste, and increase overall efficiency.
Enhancing Drug Discovery and Development
Machine learning accelerates the drug discovery and development process by identifying new drug candidates, predicting drug efficacy and safety, and streamlining the search for innovative treatments.
Identifying New Drug Candidates
Machine learning can expedite the drug discovery process by identifying potential new drug candidates more quickly and accurately. By analyzing chemical structures, biological data, and drug-target interactions, ML algorithms can predict the therapeutic potential of compounds and streamline the search for new treatments.
Predicting Drug Efficacy and Safety
Machine learning can help determine the efficacy and safety of new drugs by analyzing data from clinical trials, patient populations, and preclinical studies. By identifying potential side effects, drug interactions, and patient subgroups that may respond differently to a treatment, ML can reduce the time and cost associated with drug development and improve the overall success rate of new therapies.
Advancing Telemedicine and Remote Patient Monitoring
Machine learning supports telemedicine and remote patient monitoring by identifying at-risk patients, tracking treatment adherence, and monitoring progress, enabling timely interventions and efficient use of healthcare resources.
Identifying At-Risk Patients
Machine learning can analyze data collected from telemedicine platforms and remote patient monitoring devices to identify patients who are at risk of health complications or deterioration. By flagging these individuals for further assessment or intervention, healthcare providers can ensure timely care and prevent potentially severe health issues.
Monitoring Treatment Adherence and Progress
Machine learning can track and analyze patient data to assess treatment adherence and monitor progress over time. By identifying patterns indicative of non-adherence or suboptimal treatment response, ML can alert healthcare providers to potential issues, enabling them to adjust treatment plans or provide additional support as needed. This proactive approach leads to better patient outcomes and more efficient use of healthcare resources.
Use Cases of Machine Learning in Healthcare
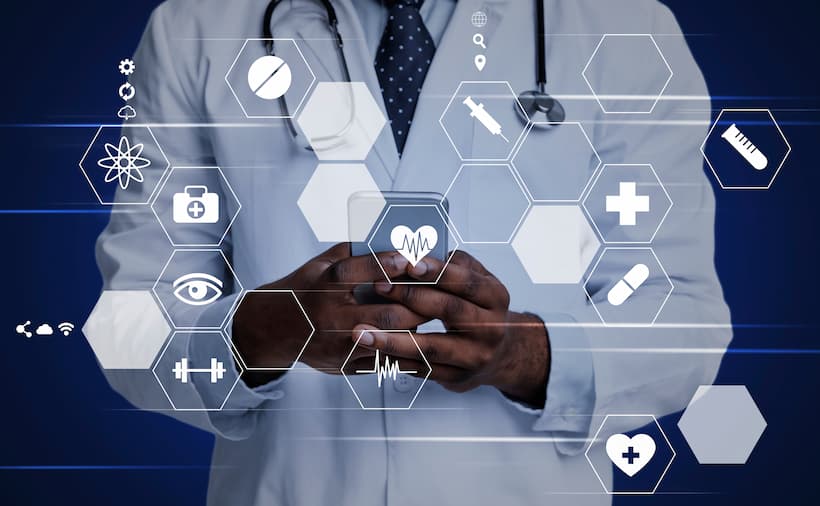
Machine learning has demonstrated immense potential in various medical specialties, addressing complex challenges and improving patient care. Here, we explore specific use cases of ML across oncology, cardiology, neurology, mental health, and infectious diseases, showcasing the transformative power of this technology.
Oncology
In oncology, machine learning contributes to early and accurate cancer detection, diagnosis, and treatment outcome prediction, helping healthcare professionals develop optimized treatment plans for better patient care.
Cancer Detection and Diagnosis
Machine learning plays a pivotal role in enhancing the accuracy of cancer detection and diagnosis. By analyzing medical images, genomic data, and electronic health records, ML algorithms can identify subtle patterns indicative of malignant tumors, often at an earlier stage than traditional diagnostic methods. Early and accurate diagnosis is crucial for improving cancer prognosis and tailoring appropriate treatment plans.
Predicting Treatment Outcomes
ML algorithms can predict treatment outcomes for cancer patients by analyzing vast amounts of clinical and molecular data. By identifying factors associated with treatment success or failure, healthcare professionals can optimize treatment strategies, minimize side effects, and improve patient outcomes.
Cardiology
Machine learning applications in cardiology focus on identifying patients at risk of cardiovascular events and personalizing treatment strategies, ultimately improving patient outcomes and overall cardiovascular health.
Identifying Patients at Risk of Cardiovascular Events
Machine learning can analyze a wide range of data sources, including electronic health records, wearable devices, and genetic information, to identify patients at risk of cardiovascular events, such as heart attacks and strokes. By detecting early warning signs, ML can enable timely interventions, reducing the incidence of life-threatening events and improving overall cardiovascular health.
Personalizing Treatment Strategies
By leveraging patient-specific data, machine learning can help cardiologists develop personalized treatment strategies for individuals with cardiovascular diseases. This tailored approach takes into account each patient’s unique risk factors, medical history, and lifestyle, leading to more effective management of heart conditions and better long-term outcomes.
Neurology
Machine learning has the potential to transform neurology by enhancing the detection and diagnosis of neurodegenerative diseases, as well as accelerating the discovery and development of novel therapies for neurological disorders.
Detecting and Diagnosing Neurodegenerative Diseases
Machine learning algorithms can analyze medical imaging data, biomarkers, and patient records to detect and diagnose neurodegenerative diseases, such as Alzheimer’s, Parkinson’s, and multiple sclerosis. Early and accurate diagnosis is critical for managing these progressive conditions, slowing their deterioration, and improving the quality of life for affected individuals.
Developing Therapies for Neurological Disorders
ML can accelerate the discovery and development of novel therapies for neurological disorders by identifying potential drug targets, predicting therapeutic efficacy, and optimizing clinical trial design. By streamlining the drug discovery process, ML can help bring effective treatments to patients faster and with reduced costs.
Mental Health
In mental health, machine learning algorithms help diagnose and treat various conditions by analyzing patient data, identifying at-risk individuals, and enabling early intervention for improved outcomes.
Diagnosing and Treating Mental Illnesses
Machine learning algorithms can analyze patient data, including speech patterns, behavioral cues, and biometric information, to diagnose and treat mental illnesses such as depression, anxiety, and bipolar disorder. By identifying patterns indicative of mental health issues, ML can facilitate early intervention and enable healthcare professionals to tailor treatments to individual needs.
Identifying At-Risk Individuals for Early Intervention
ML can analyze social media data, electronic health records, and other sources to identify individuals at risk of developing mental health issues. By flagging at-risk individuals for early intervention, machine learning can help prevent the onset of mental illnesses or mitigate their severity, ultimately improving patient outcomes and quality of life.
Infectious Diseases
Machine learning plays a crucial role in predicting, preventing, and managing infectious diseases by analyzing vast amounts of data, optimizing vaccination strategies, and guiding public health interventions.
Outbreak Prediction and Prevention
Machine learning can process vast amounts of data from disparate sources, such as climate information, population density, and travel patterns, to predict and prevent infectious disease outbreaks. By identifying areas with high outbreak potential, ML can inform public health interventions, resource allocation, and surveillance efforts, ultimately reducing the impact of infectious diseases on global health.
Guiding Vaccination Strategies
ML algorithms can analyze data on vaccine efficacy, demographics, and disease transmission to guide vaccination strategies and optimize immunization programs. By predicting the impact of various vaccination scenarios, machine learning can help public health officials make informed decisions on vaccine distribution, ultimately improving the effectiveness of vaccination campaigns and minimizing the spread of infectious diseases.
Challenges and Limitations
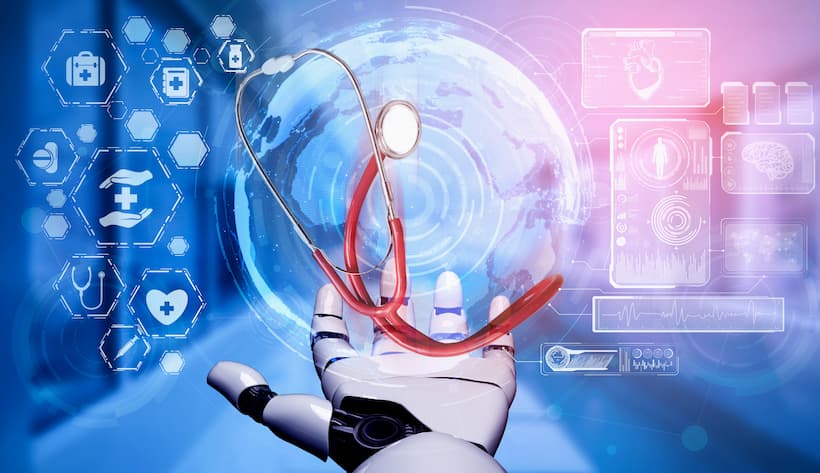
While machine learning has the potential to revolutionize healthcare, it is crucial to acknowledge the challenges and limitations associated with its implementation. These include data privacy and security concerns, issues related to data quality and representativeness, integration with existing healthcare systems, and ethical considerations. By addressing these challenges, we can harness the full potential of machine learning in healthcare while minimizing potential risks.
Data Privacy and Security Concerns
Machine learning in healthcare relies on the analysis of vast amounts of sensitive patient data. Ensuring the privacy and security of this data is of paramount importance. Healthcare organizations must implement robust security measures, such as encryption and access controls, to protect patient information from unauthorized access and data breaches.
Additionally, compliance with data protection regulations, such as HIPAA and GDPR, is essential to safeguard patient privacy and build trust in machine learning applications.
Quality and Representativeness of Data
The accuracy and effectiveness of machine learning models depend heavily on the quality and representativeness of the data used for training and validation. Incomplete, biased, or outdated data can lead to flawed predictions and suboptimal clinical decisions.
To ensure the reliability of ML algorithms, it is essential to use high-quality, diverse, and up-to-date datasets that accurately represent the target patient population. Furthermore, continuous evaluation and monitoring of ML models are necessary to maintain their performance and mitigate potential biases.
Integration with Existing Healthcare Systems
Integrating machine learning applications into existing healthcare systems can be challenging due to the complexity and heterogeneity of healthcare workflows, data formats, and information systems. Seamless integration requires the development of standardized protocols, data exchange mechanisms, and interoperable systems that facilitate communication between machine learning models and healthcare infrastructure.
Additionally, fostering collaboration between healthcare professionals, data scientists, and technology providers is essential for the successful implementation of ML in healthcare settings.
Ethical Considerations
Machine learning in healthcare raises several ethical concerns, such as fairness, transparency, and accountability. Ensuring that ML models do not perpetuate or exacerbate existing health disparities is critical. This requires the identification and mitigation of potential biases in the data and the development of algorithms that consider equity and fairness in their predictions.
Moreover, promoting transparency in the development and deployment of ML models can help establish trust and understanding among healthcare professionals and patients. Lastly, establishing clear guidelines and accountability mechanisms can help address the ethical challenges associated with the use of machine learning in healthcare.
Conclusion
In conclusion, machine learning is transforming the landscape of healthcare by offering innovative solutions to complex challenges and enhancing patient care. Through its various applications in diagnostics, personalized treatment, drug discovery, telemedicine, and public health, ML has the potential to revolutionize the way we approach healthcare and improve global health outcomes.
Despite the challenges and limitations associated with its implementation, such as data privacy, quality, integration, and ethical concerns, the future of machine learning in healthcare remains promising. By fostering continued research and development, promoting collaboration among stakeholders, and addressing the challenges, we can harness the full potential of machine learning to transform patient care and create a more accessible, efficient, and equitable healthcare system for all.
Frequently Asked Questions
1.How can machine learning help predict and manage disease outbreaks?
Machine learning algorithms can analyze large datasets from various sources, such as social media, public health records, and environmental factors, to identify patterns and trends associated with disease outbreaks. By predicting the spread of infectious diseases and providing early warnings, ML can help public health officials implement timely interventions, allocate resources effectively, and ultimately mitigate the impact of outbreaks on communities.
2.How can machine learning be used to enhance medical education and training?
Machine learning can be utilized in medical education and training by creating adaptive learning platforms, simulating complex medical scenarios, and providing personalized feedback to students. By analyzing performance data and identifying areas for improvement, ML can help medical students and healthcare professionals refine their skills, stay up-to-date with the latest medical advancements, and ultimately deliver better patient care.
3.What role does machine learning play in the development of healthcare-related robotics and automation?
Machine learning is a key component in the development of healthcare-related robotics and automation systems, such as surgical robots, robotic-assisted rehabilitation, and automated laboratory equipment. By enabling robots and automated systems to learn from data and adapt their behavior, ML can enhance the precision, efficiency, and safety of various healthcare tasks, ultimately improving patient outcomes and reducing the burden on healthcare professionals.